Building trust in AI
decisions
The TRUST Framework is an operational backbone turning Responsible AI into a measurable, actionable reality. By focusing on Transparency, Robustness, Unbiased outcomes, Security, and Testing, the TRUST Framework provides a rigorous standard for evaluating and building AI systems that inspire confidence and ensure long-term sustainability, offering a clear pathway to implement and validate Responsible AI across all parts of the ecosystem.
Recent Publications
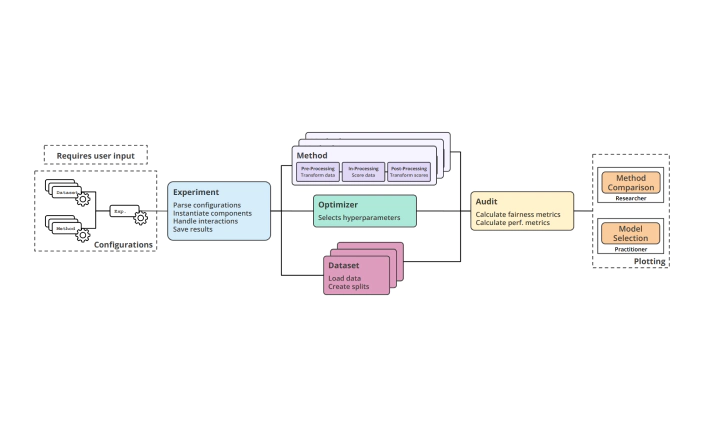
Aequitas Flow: Streamlining Fair ML Experimentation
Published at Journal of Machine Learning Research
Latest News
Deep-Graph-Sprints: Accelerated Representation Learning in Continuous-Time Dynamic Graphs paper was accepted in TMLR
Show Me What’s Wrong!: Combining Charts and Text to Guide Data Analysis paper accepted at NLVIZ a workshop at IEEE VIS 2024
Aequitas Flow: Streamlining Fair ML Experimentation paper was accepted in the journal JMLR
Cost-Sensitive Learning to Defer to Multiple Experts with Workload Constraints paper was accepted in the journal TMLR
RIFF: Inducing Rules for Fraud Detection from Decision Trees paper was accepted at RuleML+RR 2024, the leading international joint conference in the field of rule-based reasoning
Fair-OBNC: Correcting Label Noise for Fairer Datasets paper was accepted at ECAIF 2024
Show Me What’s Wrong!: Combining Charts and Text to Guide Data Analysis paper accepted at NLVIZ a workshop at IEEE VIS 2024
Aequitas Flow: Streamlining Fair ML Experimentation paper was accepted in the journal JMLR
Cost-Sensitive Learning to Defer to Multiple Experts with Workload Constraints paper was accepted in the journal TMLR
RIFF: Inducing Rules for Fraud Detection from Decision Trees paper was accepted at RuleML+RR 2024, the leading international joint conference in the field of rule-based reasoning
Fair-OBNC: Correcting Label Noise for Fairer Datasets paper was accepted at ECAIF 2024
Recent Blog Posts

Here and Now: Reusing Code at Feedzai with JupyterLab Snippets
Data scientists use different Jupyter notebooks every day — ranging from disposable ones for quick tasks to those shareable with clients.
João Palmeiro

“Show Me What’s Wrong!”: Enhancing Fraud Detection Analysis by Combining Charts and Text
Every year, millions of people fall victim to financial fraud. In 2023, the losses tied to this type of crime were estimated at US$159 billion just in the US, with some people losing all of their retirement savings to scammers.
Beatriz Feliciano
The GANfather: Using Malicious GenAI Agents to Combat Money Laundering
Digital systems have become deeply integrated into many aspects of modern life, particularly within the financial sector. While digital banking simplifies day-to-day operations for clients, it also creates new opportunities for malicious actors to exploit these systems.
Ricardo Ribeiro Pereira

Here and Now: Reusing Code at Feedzai with JupyterLab Snippets
Data scientists use different Jupyter notebooks every day — ranging from disposable ones for quick tasks to those shareable with clients.
João Palmeiro

“Show Me What’s Wrong!”: Enhancing Fraud Detection Analysis by Combining Charts and Text
Every year, millions of people fall victim to financial fraud. In 2023, the losses tied to this type of crime were estimated at US$159 billion just in the US, with some people losing all of their retirement savings to scammers.
Beatriz Feliciano
The GANfather: Using Malicious GenAI Agents to Combat Money Laundering
Digital systems have become deeply integrated into many aspects of modern life, particularly within the financial sector. While digital banking simplifies day-to-day operations for clients, it also creates new opportunities for malicious actors to exploit these systems.
Ricardo Ribeiro Pereira
Research Areas
AI Research
The AI group has a mission of building the next-gen RiskOps AI to safeguard businesses and people from fraud and financial crime that is responsible and explainable by design.
Learn More
Data Visualization
The Data Visualization group aims to better elucidate complex data for Fraud Analysts & Data Scientists with insightful beautiful data experiences.
Learn More
Systems Research
The Systems Research group aims to enhance performance & reliability of the RiskOps Platform through innovation in a number of key areas.
Learn More
AI Research
The AI group has a mission of building the next-gen RiskOps AI to safeguard businesses and people from fraud and financial crime that is responsible and explainable by design.
Learn More
Data Visualization
The Data Visualization group aims to better elucidate complex data for Fraud Analysts & Data Scientists with insightful beautiful data experiences.
Learn More
Systems Research
The Systems Research group aims to enhance performance & reliability of the RiskOps Platform through innovation in a number of key areas.
Learn More
Page printed in 11 Jul 2025. Plase see https://research.feedzai.com for the latest version.