Publication
TimeSHAP: Explaining Recurrent Models through Sequence Perturbations
Published at KDD 2021 conference, NeurIPS 2020 - HAMLETS workshop
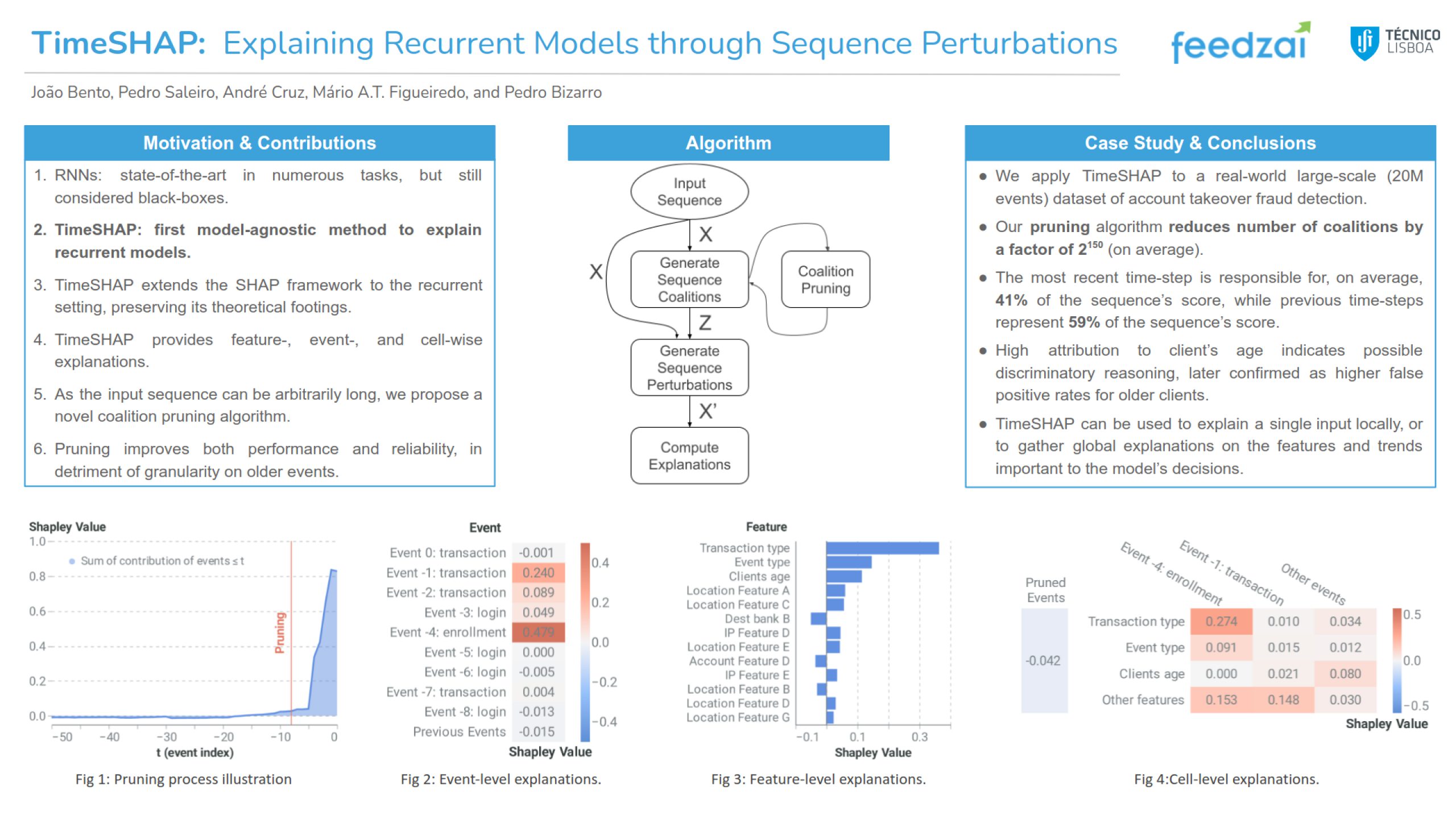
Abstract
Although recurrent neural networks (RNNs) are state-of-the-art in numerous sequential decision-making tasks, there has been little research on explaining their predictions. In this work, we present TimeSHAP, a model-agnostic recurrent explainer that builds upon KernelSHAP and extends it to the sequential domain. TimeSHAP computes feature-, timestep-, and cell-level attributions. As sequences may be arbitrarily long, we further propose a pruning method that is shown to dramatically decrease both its computational cost and the variance of its attributions. We use TimeSHAP to explain the predictions of a real-world bank account takeover fraud detection RNN model, and draw key insights from its explanations: i) the model identifies important features and events aligned with what fraud analysts consider cues for account takeover; ii) positive predicted sequences can be pruned to only 10% of the original length, as older events have residual attribution values; iii) the most recent input event of positive predictions only contributes on average to 41% of the model’s score; iv) notably high attribution to client’s age, suggesting a potential discriminatory reasoning, later confirmed as higher false positive rates for older clients.