Publication
On the Importance of Application-Grounded Experimental Design for Evaluating Explainable ML Methods
Published at AAAI-24 - Annual AAAI Conference on Artificial Intelligence
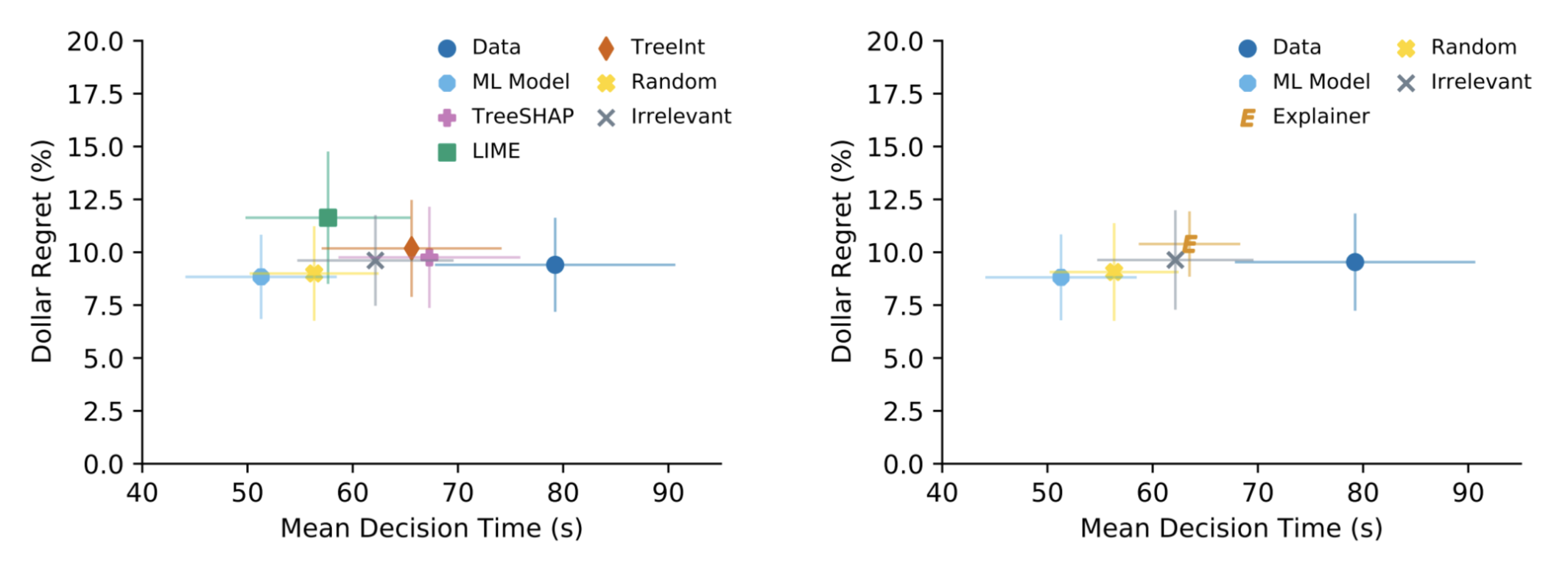
Abstract
Most existing evaluations of explainable machine learning (ML) methods rely on simplifying assumptions or proxies that do not reflect real-world use cases; the handful of more robust evaluations on real-world settings have shortcomings in their design, resulting in limited conclusions of methods’ real-world utility. In this work, we seek to bridge this gap by conducting a study that evaluates three popular explainable ML methods in a setting consistent with the intended deployment context. We build on a previous study on e-commerce fraud detection and make crucial modifications to its setup relaxing the simplifying assumptions made in the original work that departed from the deployment context. In doing so, we draw drastically different conclusions from the earlier work and find no evidence for the incremental utility of the tested methods in the task. Our results highlight how seemingly trivial experimental design choices can yield misleading conclusions, with lessons about the necessity of not only evaluating explainable ML methods using tasks, data, users, and metrics grounded in the intended deployment contexts but also developing methods tailored to specific applications. In addition, we believe the design of this experiment can serve as a template for future study designs evaluating explainable ML methods in other real-world contexts.