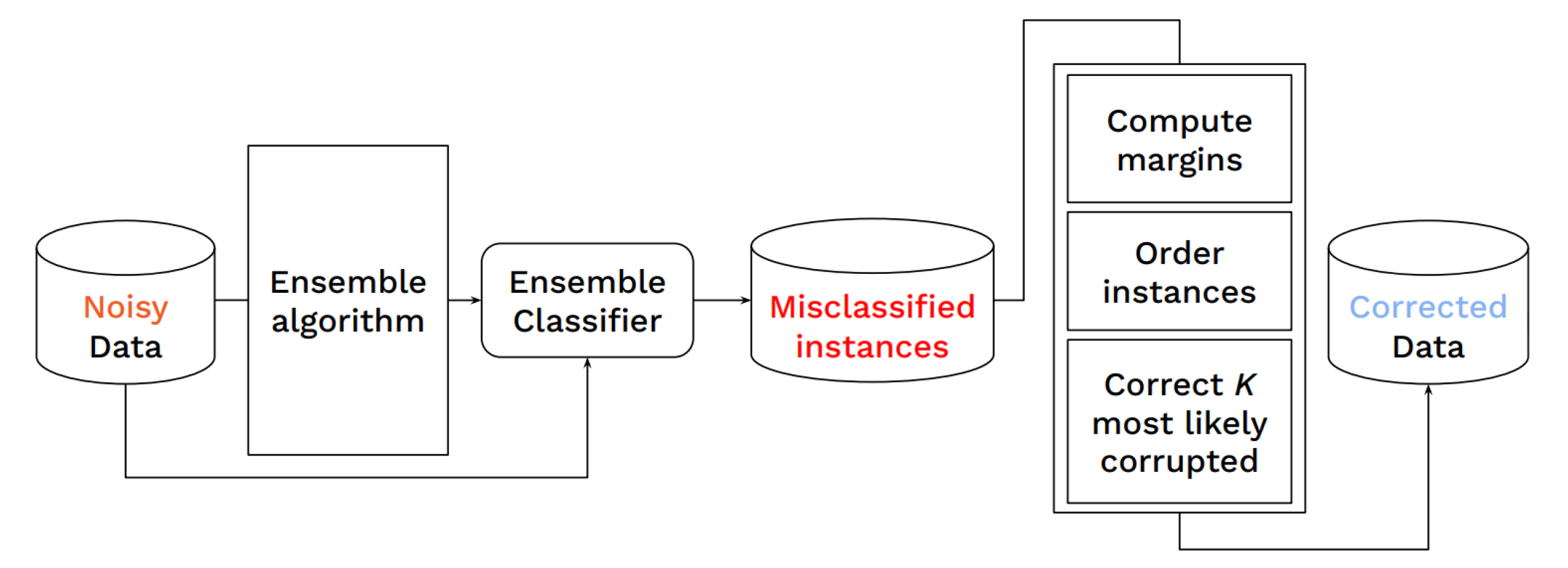
Abstract
Data used by automated decision-making systems, such as Machine Learning models, often reflects discriminatory behavior that occurred in the past. These biases in the training data are sometimes related to label noise, such as in COMPAS, where more African-American offenders are wrongly labeled as having a higher risk of recidivism when compared to their White counterparts. Models trained on such biased data may perpetuate or even aggravate the biases with respect to sensitive information, such as gender, race, or age.