Publication
Adversarial training for tabular data with attack propagation
Published at KDD Workshop on Machine Learning in Finance 2023
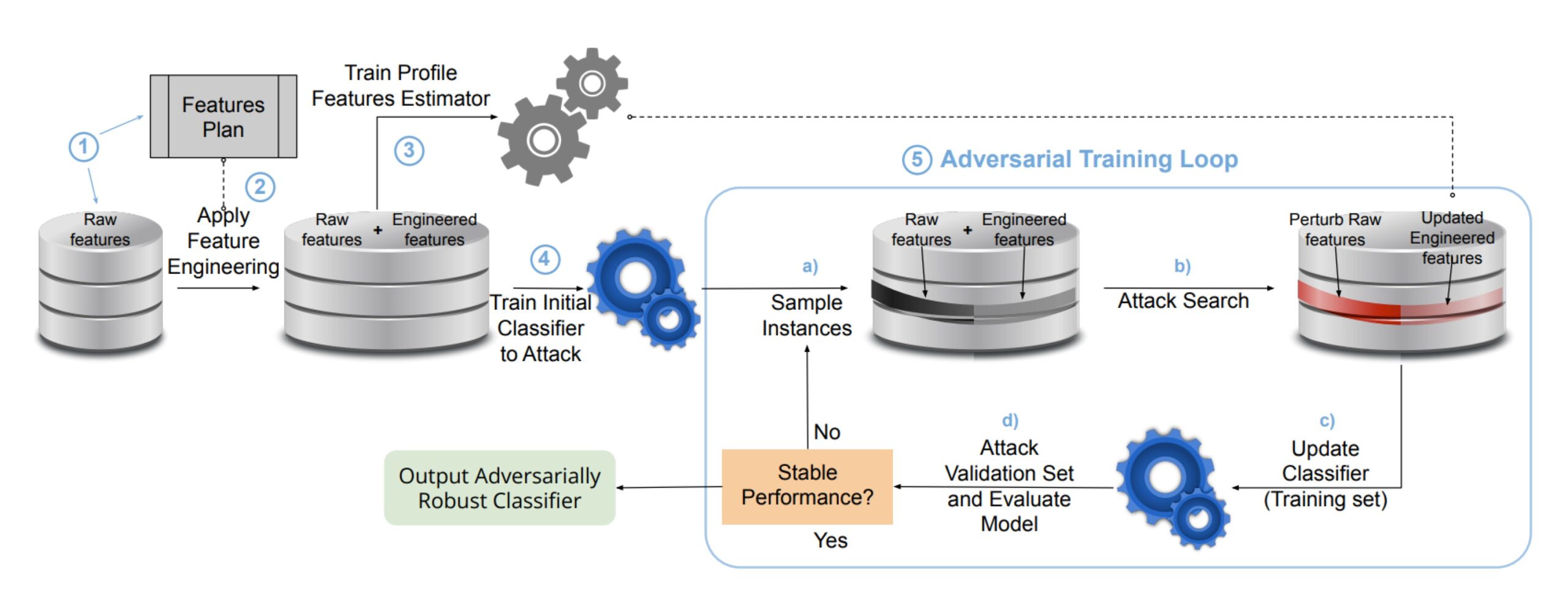
Abstract
Adversarial attacks are a major concern in security-centered applications, where malicious actors continuously try to mislead Machine Learning (ML) models into wrongly classifying fraudulent activity as legitimate, whereas system maintainers try to stop them. Adversarially training ML models that are robust against such attacks can prevent business losses and reduce the work load of system maintainers. In such applications data is often tabular and the space available for attackers to manipulate undergoes complex feature engineering transformations, to provide useful signals for model training, to a space attackers cannot access. Thus, we propose a new form of adversarial training where attacks are propagated between the two spaces in the training loop. We then test this method empirically on a real world dataset in the domain of credit card fraud detection. We show that our method can prevent about 30% performance drops under moderate attacks and is essential under very aggressive attacks, with a trade-off loss in performance under no attacks smaller than 7%.