Publication
Cost-Sensitive Learning to Defer to Multiple Experts with Workload Constraints
Published at Transactions on Machine Learning Research (07/2024)
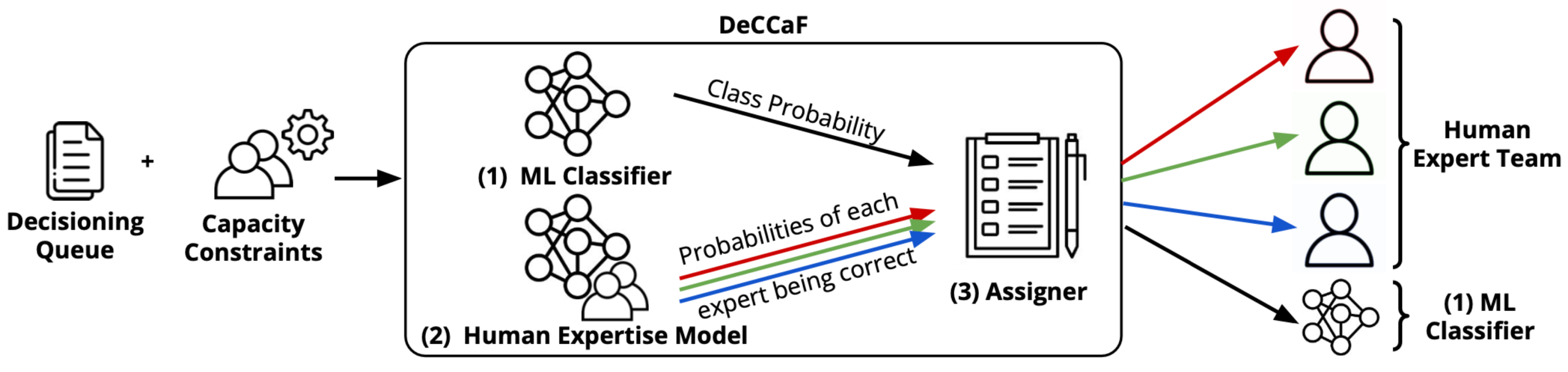
Abstract
Learning to defer (L2D) aims to improve human-AI collaboration systems by learning how to defer decisions to humans when they are more likely to be correct than an ML classifier. Existing research in L2D overlooks key real-world aspects that impede its practical adoption, namely: i) neglecting cost-sensitive scenarios, where type I and type II errors have different costs; ii) requiring concurrent human predictions for every instance of the training dataset; and iii) not dealing with human work-capacity constraints. To address these issues, we propose the deferral under cost and capacity constraints framework (DeCCaF). DeCCaF is a novel L2D approach, employing supervised learning to model the probability of human error under less restrictive data requirements (only one expert prediction per instance) and using constraint programming to globally minimize the error cost, subject to workload limitations. We test DeCCaF in a series of cost-sensitive fraud detection scenarios with different teams of 9 synthetic fraud analysts, with individual work-capacity constraints. The results demonstrate that our approach performs significantly better than the baselines in a wide array of scenarios, achieving an average 8.4% reduction in the misclassification cost. The code used for the experiments is available at https://github.com/feedzai/deccaf