Publication
FiFAR: a fraud detection dataset for learning to defer
Published at ICAIF23 - Synthetic Data for AI in Finance workshop
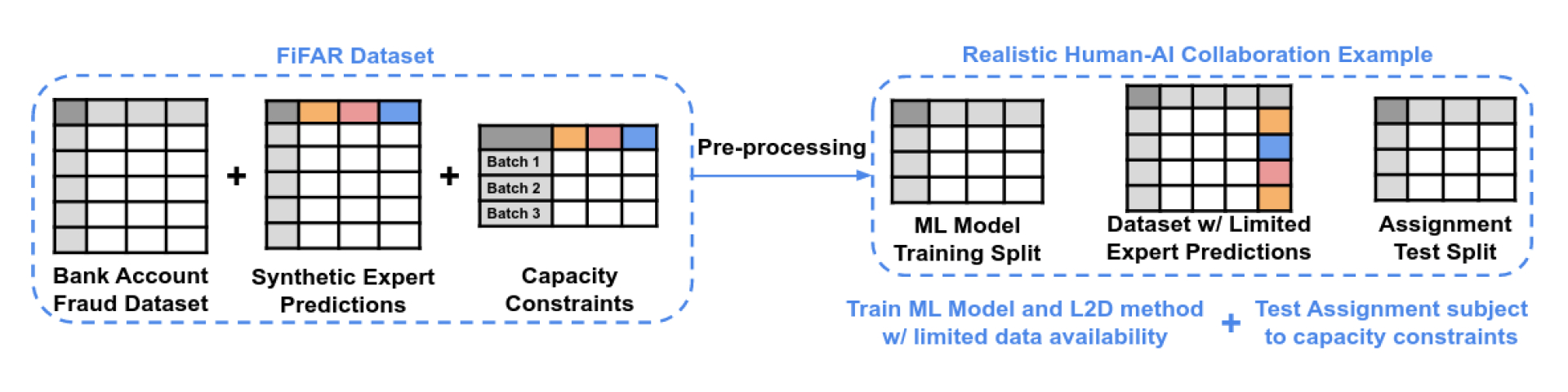
Abstract
Public dataset limitations have significantly hindered the development and benchmarking of learning to defer (L2D) algorithms, which aim to optimally combine human and AI capabilities in hybrid decision-making systems. In such systems, human availability and domain-specific concerns introduce difficulties, while obtaining human predictions for training and evaluation is costly. Financial fraud detection is a high-stakes setting where algorithms and human experts often work in tandem; however, there are no publicly available datasets for L2D concerning this important application of human-AI teaming. To fill this gap in L2D research, we introduce the Financial Fraud Alert Review Dataset (FiFAR), a synthetic bank account fraud detection dataset, containing the predictions of a team of 50 highly complex and varied synthetic fraud analysts, with varied bias and feature dependence. We also provide a realistic definition of human work capacity constraints, an aspect of L2D systems that is often overlooked, allowing for extensive testing of assignment systems under real-world conditions. We use our dataset to develop a capacity-aware L2D method and rejection learning approach under realistic data availability conditions, and benchmark these baselines under an array of 300 distinct testing scenarios. We believe that this dataset will serve as a pivotal instrument in facilitating a systematic, rigorous, reproducible, and transparent evaluation and comparison of L2D methods, thereby fostering the development of more synergistic human-AI collaboration in decision-making systems. The public dataset and detailed synthetic expert information are available at: this https URL