Publication
Weakly Supervised Multi-task Learning for Concept-based Explainability
Published at ICLR 2021 - Weakly Supervised Learning workshop
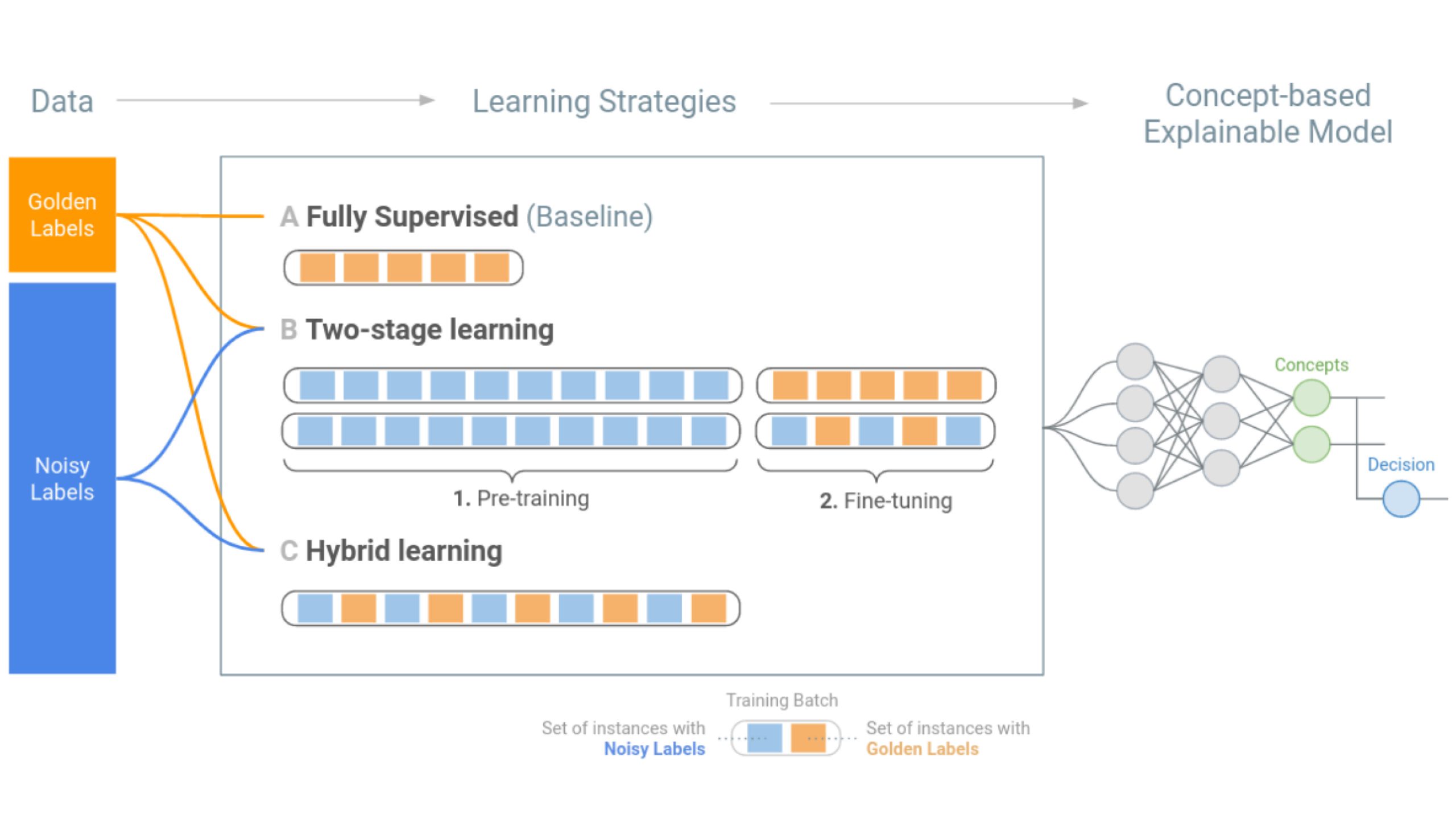
Abstract
In ML-aided decision-making tasks, such as fraud detection or medical diagnosis, the human-in-the-loop, usually a domain-expert without technical ML knowledge, prefers high-level concept-based explanations instead of low-level explanations based on model features. To obtain faithful concept-based explanations, we leverage multi-task learning to train a neural network that jointly learns to predict a decision task based on the predictions of a precedent explainability task (i.e., multi-label concepts). There are two main challenges to overcome: concept label scarcity and the joint learning. To address both, we propose to: i) use expert rules to generate a large dataset of noisy concept labels, and ii) apply two distinct multi-task learning strategies combining noisy and golden labels. We compare these strategies with a fully supervised approach in a real-world fraud detection application with few golden labels available for the explainability task. With improvements of 9.26% and of 417.8% at the explainability and decision tasks, respectively, our results show it is possible to improve performance at both tasks by combining labels of heterogeneous quality.